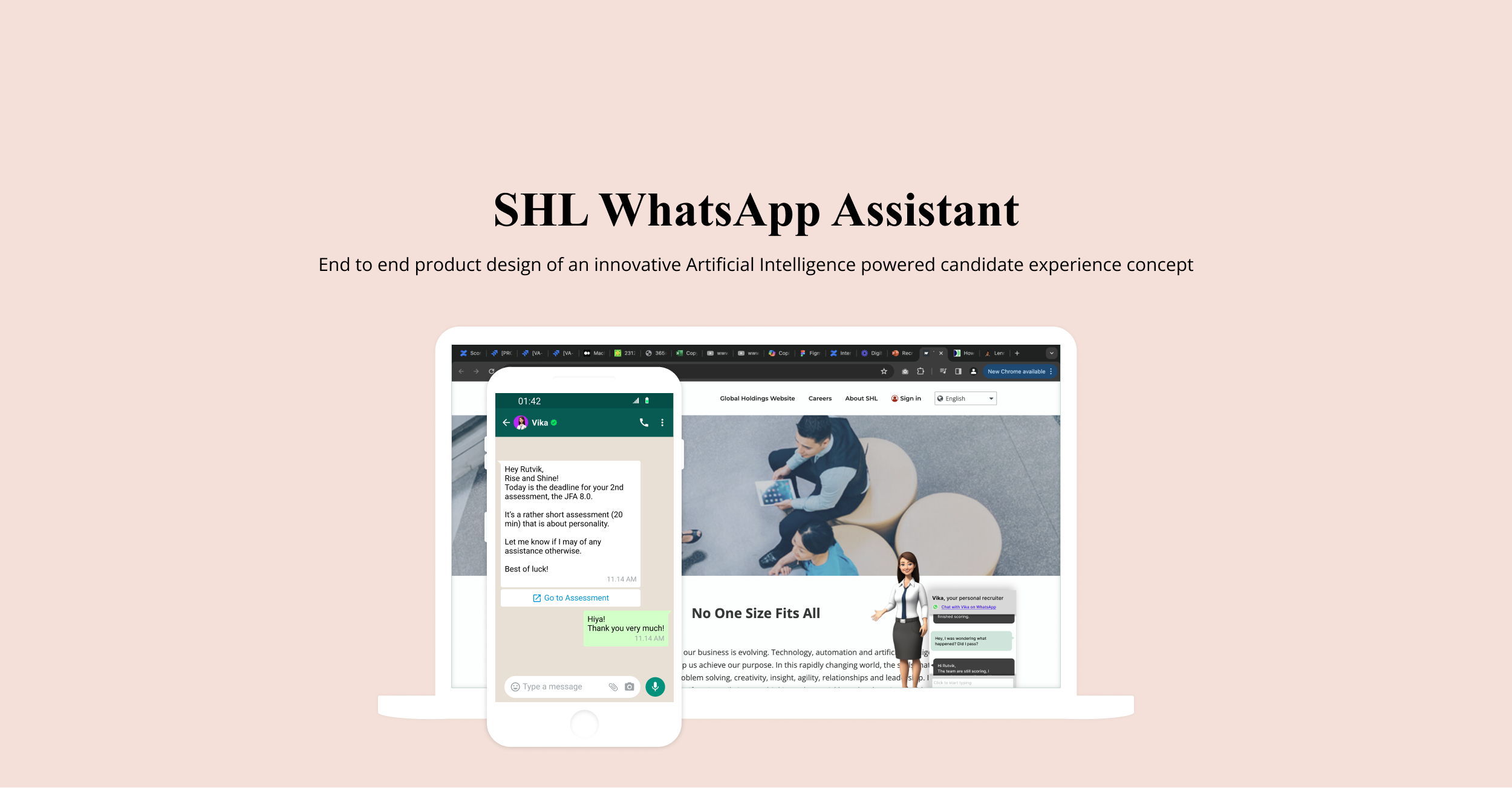
Background
SHL makes assessments to help companies recruit talent.
Candidates access the assessments via our SHL Experiences (SHLE) home page which is a micro site we build for each client with their branding etc. This page lists out the assessments which a candidate has to do and is a pretty vanilla dashboard page.
Sample screenshot to the right
Role & Duration
Role: Product Designer / Strategist | SHL
Team size: 1
(Just me)
Duration: 4 months
(Jan 2024 - Apr 2024)
Introduction
Responsibilities
Conducting market research & user research
Managing stakeholders to understand key unknowns
Conceptualising products and their vision, value proposition & Strategy
Doing feasibility studies with technical stakeholders
Creating detailed journeys, wireframes and prototypes
Communicating with executives to get budget for development
Writing stories and grooming with tech leads
Managing MVP through the entire product life cycle
Assisting Sales teams in pitching product to existing clients
Initial Problem Statement
The current SHLE dashboard (shown in section above) might have been state of the art 10 years ago but the market has evolved significantly, especially due to the emergence of generative AI.
My task was to make SHL Experiences (SHLE) better.
SHL may primarily operate in the B2B space but this page is facing the end user (candidate). Not too many, just a couple of million users per month :)
Who is the User
The users are candidates who have applied for a job in a specific company, and that company has employed SHL to administer some tests to help sift through the applicants.
Users can range from fresh graduates to seasoned professionals to managers.
The Process (This is real, I did follow it hehe xD)
Market Research
Why Pleasing Candidates is Important
Evolution of the Workforce
Candidate experience is a pivotal factor in talent acquisition. It’s a reflection of the company’s values and culture.
Positive Experience: 38% more likely to accept a job offer from company.
Negative Experience: Negative encounters are shared more readily than positive ones, influencing other candidates’ perceptions of the company.
Negative Reviews: 50% of job seekers will not even apply to a company after reading negative reviews.
Response Time: 52% of candidates have to wait 3 months or longer to receive a response from a job application.
Dropout: 60% of job seekers have abandoned a job application due to its length or complexity.
Feedback: 80% of candidates are discouraged from applying to company if they didn’t receive feedback on a previous application.
The biggest change we’ll see over the next decade is the rapid uptake of Gen Z and reduction of Boomers / Gen X.
It’s no surprise that Gen Z spend a lot of their time on social media.
What This Means
We are edging toward an attention based economy which means even higher likelihood of dropouts due to poor experiences.
Feedback: Candidates want more feedback more frequently
User Experience:
Made for mobile; not mere adaptations of desktop sites that are just barely functional but rather made specifically for mobile
Gamification; more fun and catchy. Instant gratification has shaped the candidate experience.
Personalised experienced to improve engagement
Support: Candidates want multi-channel responsive and quick support.
Accessibility: A must have for candidates with special needs.Content: With the reduced attention span, content needs to be short and snappy
Community Building: Sustainability is a key concern for the younger generation
Data & Privacy: Regulations are finally catching up and users are getting concerned about their data
Global Demand for Online Recruitment
The global online recruitment technology market size is projected to grow from $11.48 billion in 2023 to $30.87 billion by 2030, at a CAGR of 15.2%.
This is a great opportunity for us.
SHL’s Market Position
The talent acquisition process can be looked at in 2 parts;
Sourcing - Not an area of interest
There are many competitors out there, these include job boards, client websites, social media, ads etc, and are generally customer specific. Competition is much harsher here.Selection - SHL operates here
No company hires everyone that they source, they need to filter down and our assessments come in handy. We have the IO science which is our differentiator, but we are lacking in candidate experience. This chatbot might help us match the market and be more innovate. We are already well established with large clientele so have the cross sell advantage.
SWOT Analysis
Strengths - SHL’s IO science is unmatched. The large array of assessments that we have that can cater to almost all industries and roles.
Weaknesses - SHL’s candidate experience / interface (SHLE) is quite poor and does not square up with competitors
Opportunities - We have a high profile clientele allowing us to easily cross sell new solutions
Threats - Loads of competitors with really innovative candidate experiences already in the market
Takeaway
We need to refresh our candidate interface / experience (SHLE) to keep our market lead
Competitor Research
I studied some of our main competitors, focusing on their candidate interface offerings.
Target Customer & User Segments
Customer: Medium to large enterprises that do volume/Grad or professional hiring
User: Graduate / professional job applicants
User Research
Goals & Methodology
Candidate (user) and customer needs
The main goal was to learn about user needs and pains with the current candidate experience.
I had already done quite a few studies on recruiter and CHRO (buyer) personas and the talent acquisition user journeys from the client’s end so I leveraged that as a baseline.
For this project, I interviewed 5 final year students and 5 professionals in my network. These were candidates that had already applied to SHL clients and were experienced with the ‘SHLE’ dashboard which is the focus of this case study.
User Pains
I sorted and ranked the user pains on a significance scale as not all pains are equally painful hehe…
I surmised needs for candidates and customers, sourced from both the fresh interviews as well as previous studies I did.
Jobs, Pains & Gains
I finally restructured all the information I collected into jobs pains, gains for the candidate and customer.
I further split these into 3 buckets of significance, Low, Med, & High. This will come into play later.
An example of High would be “Direct complaints from clients about high dropout rates.”
From my interviews, I even got hold of an invitation email that a candidate got to complete the assessments, from one of our major clients.
Needless to say, it was terrible.
Didn’t even have a deadline; candidates had to check number of days hidden in the text, and then had to do the math in their head as to what day that will be.
There is so much wrong with this email, I don’t want to start listing everything, it will take forever.
I raised “Email Communication Refresh” as an official product backlog item, but red tape, as you are well aware, will see it get picked up in 2025, so I quickly made improvements in the copy and shared it with the relevant teams ‘unofficially’ so at least on a short term basis, the 1000 or so candidates who will see this template will have an easier life.
Prioritising Needs
I used Dan Olsen’s importance vs satisfaction framework to zoom in on needs that important and not satisfied by current alternatives.
Personas and User Journeys
I wrote 2 personas; Emma and John.
Emma was fresh graduate, Gen Z, more mobile oriented and would be mass applying to 100s of jobs
John was a professional, Millennial, more desktop oriented and would do a lot of research before applying for a select few jobs.
The distinctions in the personas above cascade into the user journeys in a significant way. These journeys were based on the current SHL candidate experience (SHLE).
I also investigated and documented the flow of information; changing information flow may have a legal impact. I kept this in mind when ideating later.
Solution Design
I documented all the stakeholders in the project and reached out to as many of them as possible, especially those nearer to the core.
Brainstorming Solutions
I ran brainstorming sessions in person and online with colleagues across the globe. I made sure to keep the jobs, pains, gains and user journeys that I created at the core of the our discussions.
I structured the discussions to ensure we tackle the following details for each idea.
Benefits to Candidates
Disadvantage to candidates
Benefits to Company / Customer
Disadvantage to Company / Customer
Cost (1-5)
Interest (1-5)
Preference = Interest / Cost
Technologies required at at high level
Prioritising solutions
We had quite a few different ideas listed in the table earlier, it wasn’t feasible to deep dive into all of them so I wanted to allocate resources smartly to the ideas that had the highest potential. To do this, I used Interest vs Cost matrix.
I shortlisted 4 solutions, In this case study, I’ll focus on solution 1; WhatsApp Chatbot.
Stakeholders
Detailed Design:
WhatsApp Chatbot
I started off by ideating on some high level journeys that the user might have with this concept.
Proposed User Journeys
Low Fidelity Wireframes
I made some wireframes to support the journeys and allow stakeholders to visualise the product.
Interviewing Stakeholders to Determine Feasibility, Costs & Risks
I put the Lo-Fi Journeys and Wireframes in front of technical stakeholders and executives to set expectations and understand what is feasible i.e., what technologies and services we will require, their costs and risks etc…
Defining scope
At this point, I was in a good position to ideate on detailed features as I had an excellent grip on the technical capabilities, and since this was an AI powered product, my academic background in Machine Learning came in handy.
Defining Features & Value Proposition
I defined 13 distinct features that would address the jobs, pains & gains that I defined earlier during my user research. I documented the features in a Value Proposition Canvas.
Prioritising Features to Define MVP
The aim was to cut the feature set and only keep what was absolutely essential for the MVP
I created a framework to prioritise features and allocate resources.
Remember that I categorised needs by significance (High, Med, Low) while conducting research.
I wrote a python script to run a weighted sum algorithm such that a feature that address 3 low level needs will be on equal footing as a feature that addressed 1 high level need. I built it such that the weights can be shifted and scaled for each project.
No such practice existed within the company so I created a template on Excel with all the relevant formulae and trained other Product team members to use it.
Here is the formula with an example:
I added all remaining features into the backlog.
Note: So far, I have created an MVP based on good research and assumptions but the final validation will come after testing with users.
Product Vision
I had continuously been updating a vision & Strategy slide for this product every week as we iterated, but now that I had defined a clear picture of MVP and features. The vision was starting to become consistent.
I used a fairly standard vision template. I shared this with executives and it gave them a very good idea of what to expect.
I devised an extensive strategy document along with the vision and If I get the time, I’ll make a whole new case study to outline the strategy of the product after a few months.
2nd Round of Workshops with Engineering
I held 2nd round workshops with engineering colleagues to validate feasibility of MVP and to understand the flow of information in detail to do high level system design.
Prioritising Needs
My product relied on Artificial Intelligence/LLM. It is widely known that companies are aggressively investing in and incorporating AI, however AI is a double edged sword in terms of marketing as some companies openly embrace it while others steer away from it.
This is due to rise in AI legislation and I know, first hand, a few clients in the US & EU regions who are steering away from using AI in anything related to recruiting. These are large market leading clients, worth more than $10 Billion, hence are not accounts that we can simply ignore.
Risk with Large Language Models (LLM)
The problem is the lack of control, as a micro possibility of the output being something untoward does exist.
Let me show a worked example, assume fault rate of 0.01% and 2,000,000 candidates. You’ve used OpenAI’s GPT 4, you know the emphirical fault rate is much higher.
That means 200 candidates could hypothetically receive faulty information.
This doesn’t sound like a big deal but the faulty information can lead to a clients being sued in a multi-million dollar lawsuits, hence it is a responsibility we take very seriously.
Architecture that I proposed
To fully eliminate the risk, I considered using a rule based chatbot instead, one that would have predetermined outputs but then the chatbot would become quite mundane.
So I proposed to have an AI model act as a contextual wrapper for the rule based chatbot.
This would give the responses a creative flare and at the same time ground the responses.
I proposed adding a 2nd AI model trained specifically in information safety to filter the output from the first. This would square the fault rate e.g., if fault rate of 1 model was 0.01% then the fault rate of my system would be 0.01%*0.01%=0.000001%.
Some clients may still not want AI for a series of other reasons, so I made a parallel version of the concept with absolutely no AI. I have shown a simplified snippet of my system diagram below.
I refreshed the system diagrams after continuous workshops with Engineering teams to add more detail. I have prepared sample conversations that would give a realistic gauge of what the 2 different versions of the product would be like.
High Fidelity Journeys & Wireframes
I created high fidelity mockups of the interface and wrote an end to end conversational journey based on the features defined earlier. PS the screenshot of the conversation I designed was 25,000 pixels longggg hence I had to convert it to a video!
Executive Presentations
Different audiences
I created 2 different powerpoint presentations for executives and other stakeholders (tech, product and commercial).
Executive Summary: 10 pages: Aimed to get executives interested and get us a budget for next steps
Product Specification: 85 pages: Contained every micro detail and rule for each feature and was aimed to be a complete guide that can be a single source of truth which can be shared with Sales, Engineering, Deployment etc…
The Outcomes
The executives were so impressed that they asked me to train other Product Managers in concept design.
I routinely run training sessions for User Research / Testing and Data analysis so I added product design as an agenda item and created some training material like structural PPT templates with key deliverables, training videos, hosted live QnA sessions etc…
All in all, I am quite proud to say I contributed significantly to the Product organisation and practices within SHL, in addition to the products themselves.
User Testing
Methodology
Testing is still underway
I am jumping through some hoops to get the authorisation from various executives, to get me access to existing live candidates.
I plan on sending out surveys to a sample of 2000 candidates via our internal survey tool.
Goals of the study:
To validate the MVP and assign value to each feature
To validate the user research on candidate needs/pains/gains that I did at the start of the project
To investigate key pains from clients e.g., high dropout rates of candidates applying to their positions.
Let’s Zoom in on Goal 3
Over multiple conversations, CHROs mentioned dropout rates are of utmost significance that the client Applicant Tracking Systems (ATS) well track. In other words, the current numbers already exist.
The first step is to quantify factors beyond the SHLE candidate interface that might be affecting dropout and figure out how much of the proverbial pie they account for. It can be done using surveys.
Hypothesis: The primary reason candidates are dropping out is because the jobs are simply not desirable.
Way to test the above hypothesis is to check dropout rate vs job desirability across different job roles and run a basic statistical test.
A sample of 2000 candidates from diverse jobs will give us a reliable estimate. I. am already liaising with relevant stakeholders to acquire the participants.
Example:
5 point scale to collect desirability of job and I can easily do a Mann-Whitney test to determine if there is a statistical difference the group that dropped out and the group that completed the assessment. Cohen’s D can tell me the practical impact of the difference.
Note that these are draft questions, the wording will change significantly before survey launch.
Another sample question is shown below that will tell us the likelihood of improvement as a result of implementing each feature. This will validate if I picked the right features for the MVP.
This WhatsApp chatbot will be a decent upgrade over the existing candidate interface and the results from the survey will give me a specific X% predicted reduction in dropout due to the implementation of each feature.
This will allow us a very targeted sale that perfectly alleviates client’s biggest pain (dropouts) which they track anyways hence will give us access to that data and we’ll be able to create benchmarks as a bonus.
Development & Launch
Currently in Planning
Keep pushing executives to get MVP on the 2024 roadmap
I’ll be the Product Manager until first release and will start handing over to a PM once official allocations are in.
I’ve already begun writing JIRA Epics for each feature
I will onboard UI designers to create client branded graphics, colour sets etc...
I’ll collaborate with Commercial teams to secure some clients for a pilot plan to hedge development costs and allow us to iterate before pitching it to higher profile clients
Once leads are available, I’ll work with Sales and Marketing teams to write pitches and give demos
I’ll write detailed Stories / Acceptance Criteria for each Epic
Request for an engineering squad and groom the stories with the tech leads
Give product demos, assist QA and complete the handover to another Product Manager
Thank you so much for reading :)
You can contact me directly at rutvik239@gmail.com or using my mobile +447432692807